在規(guī)定了各主要參數(shù)讀數(shù)分散度對(duì)汽輪機(jī)熱耗率隨機(jī)不確定度的影響s=0.1%后,根據(jù)式(5)計(jì)算得各主要參數(shù)的最小讀數(shù)次數(shù)R。某段穩(wěn)態(tài)工況的篩選過程如圖3所示。
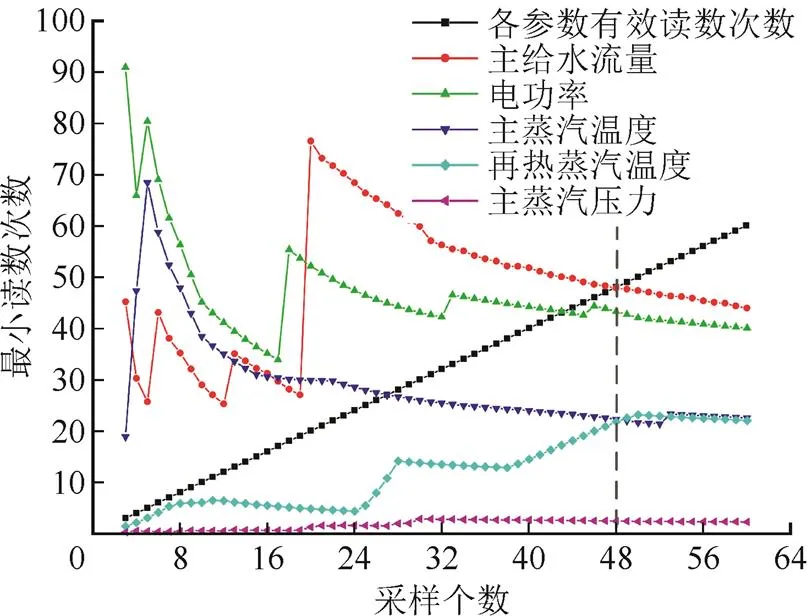
圖3 在線運(yùn)行工況下各主要參數(shù)最小讀數(shù)次數(shù)曲線
由圖3可知,電功率和主給水流量所需要的最小讀數(shù)次數(shù)最多,比其他主要參數(shù)的收斂速度慢,主要原因是電功率和主給水流量對(duì)汽輪機(jī)熱耗率的影響較大,且在實(shí)際運(yùn)行中波動(dòng)較大。由此可見,在線運(yùn)行時(shí)電功率和主給水流量的穩(wěn)定性是系統(tǒng)能否快速達(dá)到穩(wěn)態(tài)的關(guān)鍵,因此基于不確定度理論的穩(wěn)態(tài)檢測(cè)方法可以電功率和主給水流量作為系統(tǒng)處于穩(wěn)態(tài)的檢測(cè)指標(biāo)。同時(shí),各主要參數(shù)最小讀數(shù)次數(shù)R從第48個(gè)樣本后全部小于有效讀數(shù)次數(shù)a,滿足不確定度理論對(duì)多變量穩(wěn)態(tài)檢測(cè)的要求,且該穩(wěn)態(tài)區(qū)間長(zhǎng)度由穩(wěn)態(tài)檢測(cè)算法自適應(yīng)確定,由此得出該段采樣時(shí)間內(nèi)的穩(wěn)態(tài)區(qū)間是[0,48]。
3.2 穩(wěn)態(tài)檢測(cè)結(jié)果研究
以電功率和主給水流量作為穩(wěn)態(tài)檢測(cè)指標(biāo),對(duì)比機(jī)組10 h內(nèi)的穩(wěn)態(tài)檢測(cè)結(jié)果與采用滑動(dòng)窗口法的穩(wěn)態(tài)檢測(cè)結(jié)果,結(jié)果如圖4所示。圖4中,穩(wěn)態(tài)因子“1”和“0”分別代表系統(tǒng)處于穩(wěn)態(tài)和非穩(wěn)態(tài)。
由圖4可知:基于不確定度理論的穩(wěn)態(tài)檢測(cè)法得到3段穩(wěn)態(tài)區(qū)間分別是[6, 79]、[111, 168]、[301, 388],該穩(wěn)態(tài)工況區(qū)間全部落在滑動(dòng)窗口法檢測(cè)出的穩(wěn)態(tài)區(qū)間內(nèi),且能根據(jù)穩(wěn)態(tài)檢測(cè)算法自適應(yīng)地確定穩(wěn)態(tài)區(qū)間長(zhǎng)度,證明該在線穩(wěn)態(tài)檢測(cè)方法是可行的;同時(shí),在非穩(wěn)態(tài)區(qū)間[168, 301]內(nèi),雖然電功率相對(duì)穩(wěn)定,但主給水流量的波動(dòng)相對(duì)較大,本文提出的多變量穩(wěn)態(tài)檢測(cè)方法能準(zhǔn)確辨識(shí)這一微小波動(dòng),將其區(qū)間判定為非穩(wěn)態(tài),這顯然優(yōu)于滑動(dòng)窗口法的穩(wěn)態(tài)檢測(cè)結(jié)果,因此采用該穩(wěn)態(tài)檢測(cè)方法可有效提高穩(wěn)態(tài)系統(tǒng)辨識(shí)的精度和可靠性。
3.3 應(yīng)用
熱耗率是評(píng)價(jià)汽輪機(jī)性能的重要經(jīng)濟(jì)指標(biāo)。以主給水流量作為基準(zhǔn)流量,對(duì)采用該穩(wěn)態(tài)檢測(cè)算法獲得的該電廠2016年4月穩(wěn)態(tài)工況下的汽輪機(jī)熱耗率進(jìn)行計(jì)算,結(jié)果見表2。其中,將汽輪機(jī)負(fù)荷和高壓調(diào)節(jié)閥開度作為同一穩(wěn)態(tài)工況的篩選條件,電功率和熱耗率根據(jù)ASME PTC6—2004進(jìn)行了參數(shù)修正,另外還考慮了吹灰和輔助蒸汽消耗等因素的影響。由表2可知,在以上條件下所篩選出的各穩(wěn)態(tài)工況,修正后汽輪機(jī)熱耗率的平均值為7 594.8 kJ/(kW·h),不同穩(wěn)態(tài)工況下極差為37.7 kJ/(kW·h),相對(duì)偏差率為0.50%。這表明,采用基于不確定度理論的穩(wěn)態(tài)檢測(cè)算法得到的穩(wěn)態(tài)工況進(jìn)行汽輪機(jī)性能指標(biāo)計(jì)算時(shí),計(jì)算結(jié)果準(zhǔn)確度高、一致性好。
文獻(xiàn)[16]指出,當(dāng)樣本總體滿足正態(tài)分布,即~(,s2)時(shí),可根據(jù)樣本的有限總體對(duì)總體參數(shù)進(jìn)行區(qū)間估計(jì),從而得到總體參數(shù)估計(jì)值的置信區(qū)間,總體均值在置信度為95%時(shí)的置信區(qū)間為

火電機(jī)組在線運(yùn)行時(shí),影響汽輪機(jī)熱耗率的因素眾多。即使穩(wěn)態(tài)工況下相同負(fù)荷的熱耗率計(jì)算結(jié)果也會(huì)存在一定偏差。為了準(zhǔn)確估計(jì)汽輪機(jī)熱耗率包含真值的置信區(qū)間,可采用式(6)對(duì)每個(gè)月的熱耗率總體均值進(jìn)行區(qū)間估計(jì),從而得到汽輪機(jī)熱耗率隨月份的變化趨勢(shì)。
由表2計(jì)算可得,2016年4月汽輪機(jī)熱耗率在置信度為95%時(shí)置信區(qū)間為[7 583.8, 7 605.8],表明總體熱耗率真值以95%的概率落在此區(qū)間內(nèi)。同理,計(jì)算了2015年6月—2016年11月在同一總閥位、相同電功率附近汽輪機(jī)熱耗率的置信區(qū)間,得到熱耗率隨月份的變化趨勢(shì)如圖5所示。
表2 穩(wěn)態(tài)工況下汽輪機(jī)重要經(jīng)濟(jì)指標(biāo)計(jì)算結(jié)果匯總
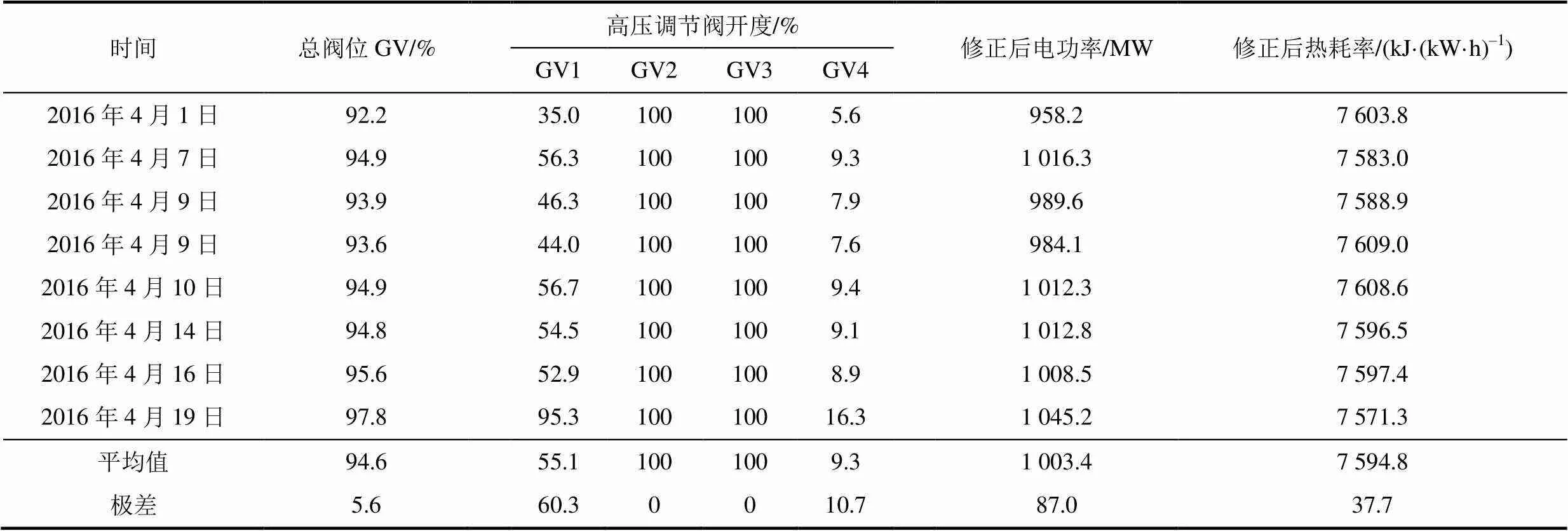
Tab.2 The calculation results summary of important economic indicators of the steam turbine under steady-state conditions
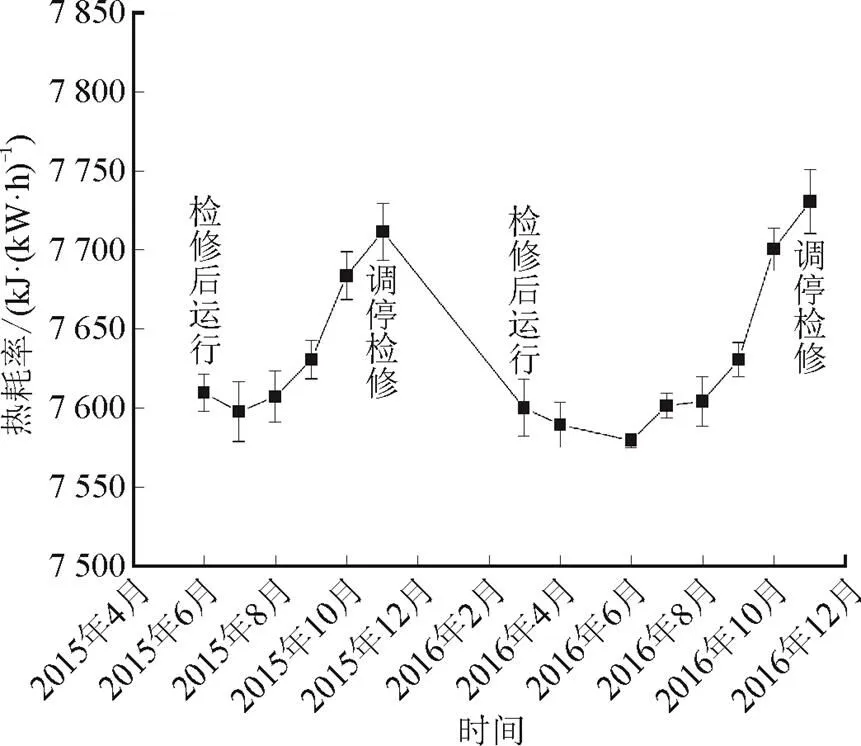
圖5 汽輪機(jī)熱耗率隨月份的變化趨勢(shì)
由圖5可見:2015年6月—2016年11月,該機(jī)組經(jīng)歷了2次調(diào)停檢修,汽輪機(jī)熱耗率總體變化趨勢(shì)為“上升-下降-上升”;在汽輪機(jī)熱耗率2次上升期(2015年9月—11月,2016年9月—11月),熱耗率分別上升約80、100 kJ/(kW·h),表明汽輪機(jī)組性能狀況惡化嚴(yán)重,因而電廠進(jìn)行了停機(jī)檢修;在熱耗率下降期(2015年11月—2016年3月),進(jìn)行了汽輪機(jī)大修和超凈排放改造,這使得汽輪機(jī)組性能提高,熱耗率降低了約110 kJ/(kW·h)。由此可知,本文提出的穩(wěn)態(tài)檢測(cè)方法計(jì)算得到的汽輪機(jī)熱耗率準(zhǔn)確反映了該機(jī)組的性能變化趨勢(shì),對(duì)汽輪機(jī)組長(zhǎng)期性趨勢(shì)化的性能監(jiān)測(cè)及調(diào)停檢修具有重要的指導(dǎo)意義。
4 結(jié) 論
1)本文從試驗(yàn)最小讀數(shù)次數(shù)計(jì)算原理出發(fā),基于不確定度理論提出了一種多變量穩(wěn)態(tài)檢測(cè)方法。該方法可將各儀表讀數(shù)分散度引起的汽輪機(jī)性能指標(biāo)計(jì)算結(jié)果隨機(jī)不確定度有效控制在規(guī)定的范圍內(nèi),具有更高的檢測(cè)靈敏度和準(zhǔn)確度,并能自適應(yīng)地確定穩(wěn)態(tài)區(qū)間長(zhǎng)度。
2)采用該穩(wěn)態(tài)檢測(cè)方法對(duì)某超超臨界1 000 MW機(jī)組歷史運(yùn)行數(shù)據(jù)進(jìn)行穩(wěn)態(tài)工況檢測(cè)。檢測(cè)結(jié)果表明,功率和主給水流量是評(píng)價(jià)熱力系統(tǒng)處于穩(wěn)態(tài)的關(guān)鍵指標(biāo),且大修后高負(fù)荷區(qū)穩(wěn)態(tài)工況下汽輪機(jī)熱耗率計(jì)算結(jié)果的平均值為7 594.8 kJ/(kW·h),不同穩(wěn)態(tài)工況下熱耗率極差為37.7 kJ/(kW·h),相對(duì)偏差率為0.50%。這表明采用基于不確定度理論的穩(wěn)態(tài)檢測(cè)算法得到的穩(wěn)態(tài)工況進(jìn)行汽輪機(jī)性能指標(biāo)計(jì)算時(shí),性能指標(biāo)計(jì)算結(jié)果準(zhǔn)確度高、一致性好。
3)該電廠2年內(nèi)高負(fù)荷區(qū)穩(wěn)態(tài)工況下汽輪機(jī)熱耗率包含真值的置信區(qū)間計(jì)算結(jié)果的變化趨勢(shì)表明:汽輪機(jī)熱耗率可準(zhǔn)確反映機(jī)組的性能惡化趨勢(shì),對(duì)汽輪機(jī)組長(zhǎng)期趨勢(shì)化的性能監(jiān)測(cè)及調(diào)停檢修具有重要的指導(dǎo)意義。
[1] 劉吉臻, 高萌, 呂游, 等. 過程運(yùn)行數(shù)據(jù)的穩(wěn)態(tài)檢測(cè)方法綜述[J]. 儀器儀表學(xué)報(bào), 2013, 34(8): 1739-1748. LIU Jizhen, GAO Meng, LV You, et al. Overview on the steady-state detection methods of process operating data[J]. Chinese Journal of Scientific Instrument, 2013, 34(8): 1739-1748.
[2] NARASIMHAN S, MAH R S H, TAMHANE A C. A composite statistical test for detecting changes of steady states[J]. Aiche Journal, 2010, 32(9): 1409-1418.
[3] NARASIMHAN S , KAO C S , MAH R S H . Detecting changes of steady states using the mathematical theory of evidence[J]. Aiche Journal, 1987, 33(11): 1930-1932.
[4] MORENO R D P. Steady state detection, data reconciliation, and gross error detection: development for industrial processes[D]. The University of New Brunswick, 2010: 2-6. http: //www. doc88. com/p-9052093220989. html.
[5] 李初福, 陳丙珍, 何小榮, 等. 用于含過失誤差數(shù)據(jù)穩(wěn)態(tài)檢測(cè)的改進(jìn)濾波法[J]. 清華大學(xué)學(xué)報(bào)(自然科學(xué)版), 2004, 44(9): 1160-1162.LI Chufu, CHEN Bingzhen, HE Xiaorong, et al. Inproved filtering method for steady state tests with measurements containing gross error[J]. Journal of Tsinghua University: Natural Science Edition, 2004, 44(9): 1160-1162.
[6] 陳文馳, 劉飛. 一種改進(jìn)的基于多項(xiàng)式濾波的穩(wěn)態(tài)檢測(cè)方法[J]. 控制工程, 2012, 19(2): 195-197. CHEN Wenchi, LIU Fei. An improved steady-state identification method based on polynomial filtering[J]. Control Engineering of China, 2012, 19(2): 195-197.
[7] 付克昌, 戴連奎, 吳鐵軍. 基于多項(xiàng)式濾波算法的自適應(yīng)穩(wěn)態(tài)檢測(cè)[J]. 化工自動(dòng)化及儀表, 2006, 33(5): 18-22. FU Kechang, DAI Liankui, WU Tiejun. Method of adaptive steady state detection based on polynomial filtering[J]. Control and Instruments in Chemical Industry, 2006, 33(5): 18-22.
[8] 陳玉玲, 徐聿晟, 沈珂敏, 等. 基于過程系統(tǒng)測(cè)量數(shù)據(jù)的穩(wěn)態(tài)檢測(cè)與數(shù)據(jù)處理方法[J]. 化工自動(dòng)化及儀表, 2013, 40(2): 222-225. CHEN Yuling, XU Yusheng, SHEN Kemin, et al. Steady state detection and data processing based on measurement data of process system[J]. Control and Instruments in Chemical Industry, 2013, 40(2): 222-225.
[9] 劉吉臻, 楊光軍, 譚文. 一種基于數(shù)據(jù)驅(qū)動(dòng)的電站狀態(tài)穩(wěn)定性能評(píng)價(jià)指標(biāo)[J]. 熱力發(fā)電, 2008, 37(1): 45-49. LIU Jizhen, YANG Guangjun, TAN Wen. An index based data-driven for evaluating the state stability in power plant[J]. Thermal Power Generation, 2008, 37(1): 45-49.
[10] JIANG T, CHEN B, HE X, et al. Application of steady-state detection method based on wavelet transform[J]. Computers & Chemical Engineering, 2003, 27(4): 569-578.
[11] 饒宛, 方立軍, 張晗, 等. 基于Fisher有序聚類的汽輪機(jī)試驗(yàn)數(shù)據(jù)穩(wěn)態(tài)檢測(cè)方法[J]. 電站系統(tǒng)工程, 2016, 32(1): 64-66.RAO Wan, FANG Lijun, ZHANG Han, et al. Steady state detection of turbine performance test data based on fisher ordered clustering method[J]. Power System Engineering, 2016, 32(1): 64-66.
[12] 畢小龍, 王洪躍, 司風(fēng)琪, 等. 基于趨勢(shì)提取的穩(wěn)態(tài)檢測(cè)方法[J]. 動(dòng)力工程, 2006, 26(4): 503-506. BI Xiaolong, WANG Hongyue, SI Fengqi, et al. A method based on tendency distillation for ascertaining state steadiness[J]. Journal of Power Engineering, 2006, 26(4): 503-506.
[13] 呂游, 劉吉臻, 趙文杰, 等. 基于分段曲線擬合的穩(wěn)態(tài)檢測(cè)方法[J]. 儀器儀表學(xué)報(bào), 2012, 33(1): 194-200. LV You, LIU Jizhen, ZHAO Wenjie, et al. Steady state detecting method based on piecewise curve fitting[J]. Chinese Journal of Scientific Instrument, 2012, 33(1): 194-200.
[14] Test uncertainty: ASME PTC19. 1—2005[S]. New York: The American Society of Mechanical Engineers, 2006: 5.
[15] Steam turbines performance test codes: ASME PTC—2004[S]. New York: The American Society of Mechanical Engineers, 2005: 12-13.
[16] 施雨. 應(yīng)用數(shù)理統(tǒng)計(jì)[M]. 西安: 西安交通大學(xué)出版社, 2013: 58-60. SHI Yu. Apply mathematical statistics[M]. Xi’an: Xi’an Jiaotong University Press, 2013: 58-60.
A steady state detection method based on uncertainty theory and its application
YANG Li, JIANG Hao, JING Xinjing, ZENG Lifei
(Xi’an Thermal Power Research Institute Co., Ltd., Xi’an 710054, China)
On the basis of the uncertainty theory, a multi-variable steady-state detection method is proposed. This method extracts steady state information by controlling the influence of each main operating parameter on random uncertainty of the performance index within a specified limits, which can adaptively determine the steady-state interval length. Moreover, this method is applied to carry out steady-state detection for operating data of an ultra-supercritical 1 000 MW thermal power unit. The results show that, the power and feed water flow are key indicators for the system’s steady state evaluation, the performance index calculated by the detected steady state condition has high accuracy and good consistency, which can be applied for performance monitoring and diagnosis of steam turbines. By comparing the steady-state detection results with the sliding window method, it finds that this method has higher detection sensitivity and accuracy. Therefore, the steady state detection method proposed in this paper lays a foundation for thermal power units’ performance monitoring and evaluation, fault detection and diagnosis by using historical operational data, which alleviates many problems faced by current steam turbines’ thermal performance tests and has certain engineering application values.
thermal power unit, steam turbine, steady-state detection, uncertainty, the minimum number of readings, performance calculation, heat consumption rate, confidence interval
TK267; TP274
A
10.19666/j.rlfd.201812234
楊利, 江浩, 井新經(jīng), 等. 基于不確定度理論的穩(wěn)態(tài)檢測(cè)方法及其應(yīng)用[J]. 熱力發(fā)電, 2019, 48(5): 139-144. YANG Li, JIANG Hao, JING Xinjing, et al. A steady state detection method based on uncertainty theory and its application[J]. Thermal Power Generation, 2019, 48(5): 139-144.
2018-12-27
楊利(1990—),男,碩士研究生,主要研究方向?yàn)殡姀S節(jié)能與診斷,yangli@tpri.com.cn。
(責(zé)任編輯 劉永強(qiáng))